[ad_1]
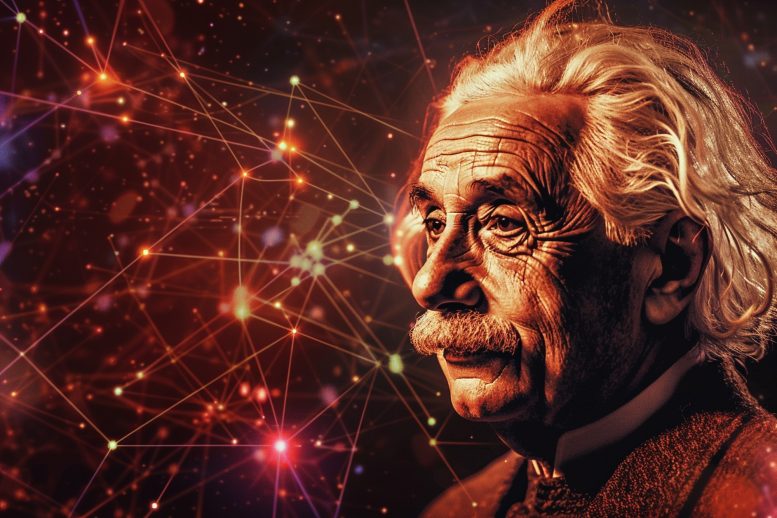
Researchers at Forschungszentrum Jülich have developed an AI able to formulating bodily theories by recognizing patterns in advanced knowledge units, a feat traditionally achieved by nice physicists like Isaac Newton and Albert Einstein. This AI, a part of the “Physics of AI” initiative, simplifies advanced interactions in knowledge to develop new theories, differing from standard approaches by making the theories explainable and grounded within the language of physics. Credit score: SciTechDaily.com
The event of a brand new principle is usually related to the greats of physics. You may consider Isaac Newton or Albert Einstein, for instance. Many Nobel Prizes have already been awarded for brand spanking new theories. Researchers at Forschungszentrum Jülich have now programmed a man-made intelligence that has additionally mastered this feat.
Their AI is ready to acknowledge patterns in advanced knowledge units and to formulate them in a bodily principle. The event of a brand new principle is usually related to the greats of physics. You may consider Isaac Newton or Albert Einstein, for instance. Many Nobel Prizes have already been awarded for brand spanking new theories. Researchers at Forschungszentrum Jülich have now programmed a man-made intelligence that has additionally mastered this feat. Their AI is ready to acknowledge patterns in advanced knowledge units and to formulate them in a bodily principle.
Within the following interview, Prof. Moritz Helias from Forschungszentrum Jülich’s Institute for Superior Simulation (IAS-6) explains what the “Physics of AI” is all about and to what extent it differs from standard approaches.
How do physicists provide you with a brand new principle?
You often begin with observations of the system earlier than trying to suggest how the completely different system parts work together with one another as a way to clarify the noticed conduct. New predictions are then derived from this and put to the check. A widely known instance is Isaac Newton’s regulation of gravitation. It not solely describes the gravitational pressure on Earth, nevertheless it can be used to foretell the actions of planets, moons, and comets – in addition to the orbits of recent satellites – pretty precisely.
Nevertheless, the best way during which such hypotheses are reached all the time differs. You can begin with basic ideas and fundamental equations of physics and derive the speculation from them, or you may select a phenomenological strategy, limiting your self to describing observations as precisely as doable with out explaining their causes. The issue lies in deciding on a great strategy from the quite a few approaches doable, adapting it if needed, and simplifying it.
What strategy are you taking with AI?
Generally, it entails an strategy referred to as “physics for machine studying”. In our working group, we use strategies of physics to research and perceive the advanced perform of an AI.
The essential new thought developed by Claudia Merger from our analysis group was to first use a neural community that learns to precisely map the noticed advanced conduct to an easier system. In different phrases, the AI goals to simplify all of the advanced interactions we observe between system parts. We then use the simplified system and create an inverse mapping with the educated AI. Getting back from the simplified system to the advanced one, we then develop the brand new principle. On the best way again, the advanced interactions are constructed up piece by piece from the simplified ones. In the end, the strategy is subsequently not so completely different from that of a physicist, with the distinction being that the best way during which the interactions are assembled is now learn from the parameters of the AI. This attitude on the world – explaining it from interactions between its numerous components that observe sure legal guidelines – is the idea of physics, therefore the time period “physics of AI”.
Wherein functions was AI used?
We used a knowledge set of black and white photos with handwritten numbers, for instance, which is commonly utilized in analysis when working with neural networks. As a part of her doctoral thesis, Claudia Merger investigated how small substructures within the photos, similar to the sides of the numbers, are made up of interactions between pixels. Teams of pixels are discovered that are usually brighter collectively and thus contribute to the form of the sting of the quantity.
How excessive is the computational effort?
The usage of AI is a trick that makes the calculations doable within the first place. You in a short time attain a really massive variety of doable interactions. With out utilizing this trick, you can solely take a look at very small programs. Nonetheless, the computational effort concerned remains to be excessive, which is because of the truth that there are numerous doable interactions even in programs with many parts. Nevertheless, we will effectively parameterize these interactions in order that we will now view programs with round 1,000 interacting parts, i.e. picture areas with as much as 1,000 pixels. Sooner or later, a lot bigger programs must also be doable via additional optimization.
How does this strategy differ from different AIs similar to ChatGPT?
Many AIs purpose to be taught a principle of the info used to coach the AI. Nevertheless, the theories that the AIs be taught often can’t be interpreted. As an alternative, they’re implicitly hidden within the parameters of the educated AI. In distinction, our strategy extracts the discovered principle and formulates it within the language of interactions between system parts, which underlies physics. It thus belongs to the sphere of explainable AI, particularly the “physics of AI”, as we use the language of physics to elucidate what the AI has discovered. We are able to use the language of interactions to construct a bridge between the advanced interior workings of AI and theories that people can perceive.
Reference: “Studying Interacting Theories from Knowledge” by Claudia Merger, Alexandre René, Kirsten Fischer, Peter Bouss, Sandra Nestler, David Dahmen, Carsten Honerkamp and Moritz Helias, 20 November 2023, Bodily Evaluate X.
DOI: 10.1103/PhysRevX.13.041033
[ad_2]
Supply hyperlink